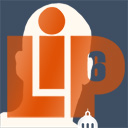
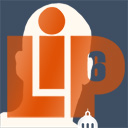
Équipe : MOCAH - Modèles et Outils en ingénierie des Connaissances pour l'Apprentissage Humain
Axe : AID (👥👥).Co-Responsables :
Amel Yessad Campus Pierre et Marie Curie 26-00/307
François Bouchet Campus Pierre et Marie Curie 26-00/305
Brève présentation
L'équipe MOCAH (Modèles et Outils en ingénierie des Connaissances pour l'Apprentissage Humain) est spécialisée dans l'utilisation de technologies fondées sur l'IA (ingénierie des connaissances, ontologies, agents conversationnels, fouille de données...) pour l'aide à l'apprentissage humain. L'essentiel de nos travaux visent à proposer des modèles intégrant les connaissances humaines dans des systèmes d'aide à l'apprentissage afin d'améliorer les modèles de l'apprenant, le diagnostic, le feedback et les systèmes eux-mêmes. Ces connaissances peuvent être collectées : (1) directement auprès des humains (experts, enseignants, apprenants...) et/ou (2) extraites des données à l'aide de techniques de fouilles de données éducatives. Les modèles et outils sont associés à des environnements informatiques ouverts tels que des simulations, des jeux sérieux et des systèmes de réalité virtuelle, c’est-à-dire des environnements hautement interactifs. Nous nous intéressons également à d'autres environnements moins interactifs tels que les MOOCs pour la quantité de données qu'ils procurent afin d’étudier les problématiques liées à l'évaluation, le retour aux apprenants et l'adaptation de scénarios pédagogiques. L'équipe MOCAH est orientée vers la recherche à visée applicative, cruciale dans le contexte de l'apprentissage assisté par la technologie afin de développer des applications concrètes et les tester dans un contexte écologique (en classe et non seulement en laboratoire), et interdisciplinaire
Environnements Informatiques pour l'Apprentissage Humain (EIAH), Intelligence artificiel pour l'apprentissage, learning analytics, serious games, E-learning, E-formation, TICE, ressources pédagogiques, outils auteurs, modélisation cognitive, diagnostic de compétences, adaptation, apprentissage par le jeu, métadonnées,
Aucune manisfestation prévue actuellement.
ArchivesSélection de publications
- Ö. Yalçin, S. Lallé, C. Conati : “An Intelligent Pedagogical Agent to Foster Computational Thinking in Open-Ended Game Design Activities”IUI '22: 27th International Conference on Intelligent User Interfaces, Helsinki, Finland, pp. 633-645, (ACM)[Yalçin 2022]
- J. Hernandez, M. Muratet, M. Pierotti, Th. Carron : “Can We Detect Non-playable Characters’ Personalities Using Machine And Deep Learning Approaches?”European Conference on Game Based Learning, vol. 16 (1), Lisbonne, Portugal, pp. 271-279[Hernandez 2022a]
- A. Yessad : “Personalizing the Sequencing of Learning Activities by using the Q-Learning and the Bayesian Knowledge Tracing”17th European Conference on Technology-Enhanced Learning, Toulouse, France[Yessad 2022b]
- K. Oliver‑Quelennec, F. Bouchet, Th. Carron, K. Fronton Casalino, C. Pinçon : “Adapting Learning Analytics Dashboards by and for University Students”Educating for a New Future: Making Sense of Technology-Enhanced Learning Adoption, vol. 13450, Lecture Notes in Computer Science, Toulouse, France, pp. 299-309, (Springer International Publishing), (ISBN: 978-3-031-16290-9)[Oliver-Quelennec 2022c]
- E. Ferrier‑Barbut, Ph. Gauthier, V. Luengo, G. CANLORBE, M.‑A. Vitrani : “Measuring the quality of learning in a human-robot collaboration: a study of laparoscopic surgery”ACM Transactions on Human-Robot Interaction, (ACM)[Ferrier-Barbut 2022]
- R. Chaker, F. Bouchet, R. Bachelet : “How do online learning intentions lead to learning outcomes? The mediating effect of the autotelic dimension of flow in a MOOC”Computers in Human Behavior, vol. 134, pp. 107306, (Elsevier)[Chaker 2022]
- C. Canellas, F. Bouchet, Th. Arribe, V. Luengo : “Towards Learning Analytics Metamodels in a Context of Publishing”Proceedings of the 13th International Conference on Computer Supported Education, vol. 2, Prague (virtual), Czechia, pp. 45-54, (SciTePress)[Canellas 2021]
- M. Muratet, D. Garbarini : “Accessibility and serious games: What about Entity- Component-System software architecture?”GALA 2020, Laval, France[Muratet 2020a]
- F. Harrak, F. Bouchet, V. Luengo : “From Student Questions to Student Profiles in a Blended Learning Environment”Journal of Learning Analytics, vol. 6 (1), pp. 54-84[Harrak 2019c]
- Th. Carron, G. Houzet, H. Abed, Ph. Pernelle, P.‑J. Lainé, S. Talbot : “Teaching Digital Literacy: The Outcomes from a Learning Lab”Journal of Electrical Engineering, vol. 6 (2), pp. 75-84, (David Publishing Company)[Carron 2018]
- Y. Bourrier, J. Francis, C. Garbay, V. Luengo : “A Hybrid Architecture for Non-Technical Skills Diagnosis”Intelligent Tutoring Systems (ITS 2018), vol. 10858, Lecture Notes in Computer Science, Montreal, Canada, pp. 300-305, (Springer)[Bourrier 2018b]
- F. Harrak, F. Bouchet, V. Luengo, P. Gillois : “Profiling Students from Their Questions in a Blended Learning Environment”LAK '18 Proceedings of the 8th International Conference on Learning Analytics and Knowledge, Sydney, Australia, pp. 102-110, (ACM)[Harrak 2018]
- J. Alvarez, J.‑Y. Plantec, M. Vermeulen, Ch. Kolski : “RDU Model dedicated to evaluate needed counsels for Serious Game projects”Computers and Education, vol. 114, pp. 38-56, (Elsevier)[Alvarez 2017]
- B. Monterrat, A. Yessad, F. Bouchet, E. Lavoué, V. Luengo : “MAGAM: A Multi-Aspect Generic Adaptation Model for Learning Environments”Data Driven Approaches in Digital Education, vol. 10474, Lecture Notes in Computer Science, Tallinn, Estonia, pp. 139-152, (Springer International Publishing)[Monterrat 2017b]
Contact
vanda.luengo (at) nulllip6.fr