Séminaire Donnees et APprentissage Artificiel
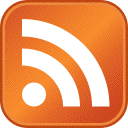
Feature Selection as a One-Player Game
Wednesday, July 7, 2010Speaker(s) : Romaric Gaudel (LRI, Université Paris-Sud)
This paper formalizes Feature Selection as a Reinforcement Learning problem, leading to a provably optimal though intractable selection policy. As a second contribution, this paper presents an approximation thereof, based on a one-player game approach and relying on the Monte-Carlo tree search UCT (Upper Confidence Tree) proposed by Kocsis and Szepesvari (2006). The Feature Uct SElection (FUSE) algorithm extends UCT to deal with i) a finite unknown horizon (the target number of relevant features); ii) the huge branching factor of the search tree, reflecting the size of the feature set. Finally, a frugal reward function is proposed as a rough but unbiased estimate of the relevance of a feature subset. A proof of concept of FUSE is shown on benchmark data sets.
More details here …
Thomas.Baerecke (at) nulllip6.fr