Séminaire Donnees et APprentissage Artificiel
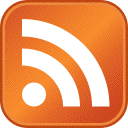
Semi-supervised learning in huge graphs
Tuesday, November 25, 2008Speaker(s) : Marco Gori (University of Siena)
Beginning from studies on the inverse problem, I review the classic variational framework to learning that is based on the minimization of a loss function and on a regularization term that penalizes abrupt changes. Then, I’ll address mainly the critical issue of the lack of labeled examples that affect most challenging real-world problems. Amongst the solutions that have been proposed to face this relevant issue, some of the most challenging ones make use of a graphical representations of the examples with links expressing binary relationships. It has been shown that collective classification schemes can be carried out as a result of a variational approach taking place in the discrete graphical setting. However, these kinds of learning schemes are transductive in nature and can only operate whenever the diffusion of the information takes place on a huge, yet static graph. I this talk I show how a more general variational framework in the continuum setting can incorporate the mentioned transductive schemes and, most importantly, can operate by a truly semi-supervised scheme also on examples that are not linked to the graph.
More details here …
Thomas.Baerecke (at) nulllip6.fr