Séminaire Complex Networks
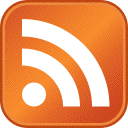
Motifs Distribution in Exchangeable Random Networks
Vendredi 28 février 2014Intervenant(s) : Pierre-André Maugis (University College London)
In this talk I will show how the relationship between the local and global characteristics of random graphs can be used for statistical inference.
There exists a long history of research on graphs/networks as mathematical objects. However, the need for methods allowing for statistical inference based on network data is but recent, and was prompted by the current boom in available network datasets along with their relevance to research in the social and biological sciences.
The problem we face, set in the classical statistical paradigm, consists in seeing the networks as issuing from a random process, and in trying to infer from the observed network some characteristics of the said random process. The difficulty is both theoretical and practical: we only observe one realisation of the network (where statisticians usually assume they have a large number of repeated measurements), and networks are large objects, easily involving millions of connections, which raises computational issues.
Studying networks through the local characteristics that are motifs (e.g. triangles, squares, cliques, ...) offers a solution to both problems at once. Motifs are small (and hence computationally amenable), and occur multiple times throughout the network. Moreover, as we will show, under the assumption of exchangeability one can relate the random process from which the network ensued and the distribution of realised motifs. Using these results we will describe how one can use motifs to produce sound statistical inference on network data.
This is a joint Work with Sofia Olhede and Patrick Wolfe.
Plus d'informations ici …
francois.queyroi (at) nulllip6.fr