Séminaire Donnees et APprentissage Artificiel
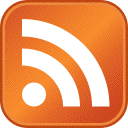
Intelligent Symbolic Clustering through High Dimensional Space
Vendredi 8 avril 2011Intervenant(s) : Mika Sato-Ilic (University of Tsukuba, Japan)
Today, we are faced with the challenge of analyzing vast amounts of high dimensional data and fuzzy cluster analysis is a well known exploratory data analysis used across a broad range of scientific areas. Fuzzy cluster analysis obtains the fuzzy classification structure in the data space which reflects the pervasiveness of imprecision and uncertainty which exists in the real world.
In this presentation, several methodologies that exploit the fuzzy classification structure in higher dimensional data space will be presented in order to demonstrate how a more accurate result may be obtained. Specifically, two points of view will be discussed. Firstly, obtaining of a more efficient result in the lower dimensional space which is a summarization of the original high dimensional data structure by using the fuzzy clustering result obtained in the higher dimensional space. Secondly, using objects space which is a higher dimensional space then the original data space. The inner product space mapped from the original data space is used to increase the value of the partition coefficient for the classification for noisy data.
Concerning the first point of view, I will present a principal component analysis (PCA) exploiting the fuzzy classification structure in high dimensional space. The similarity structure of objects in a high dimensional space is extracted by using a fuzzy clustering method, and by introducing the result to the PCA. A new PCA is proposed considering the similarity structure of objects in a high dimensional space in order to obtain a more accurate result of the PCA.
From the second point of view, I will present a generalized nonlinear fuzzy clustering model using similarity data with various structures. The generalized operators are defined on a product space of linear spaces. A kernel fuzzy clustering model is a special case of this model in which the degree of objects to clusters is estimated in a mapped higher dimensional space using kernel functions. In other words, similarity in a higher dimensional space than the data space is used in order to measure the degree of similarity between a pair of objects.
I believe that the adaptation of fuzzy clustering result is an effective means to achieve a breakthrough. An ideal solution for analysis is the capture of unique features of data with the intention of discovering uncertainty through the use of a set of robust and modern methods, so to this end, analyses with fuzzy clustering are proposed.
Plus d'informations ici …
Sahar.Changuel (at) nulllip6.fr